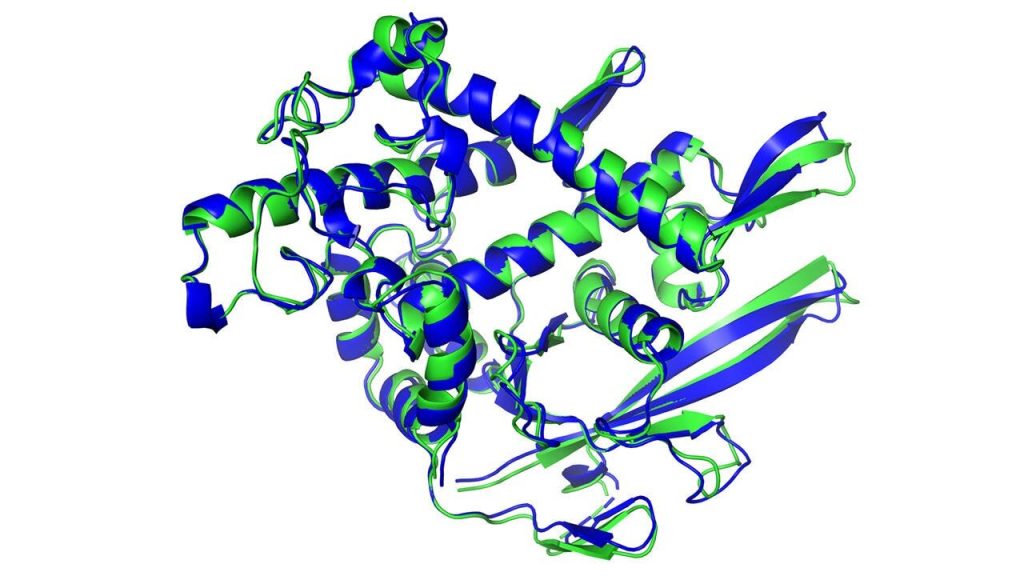
Last December when 2020 neared its end, we had finally received some hopeful news: the first COVID vaccine was accepted by the EU. It felt like the development of a proper vaccine took ages, which resulted in us finally having a bright spot on the horizon after hearing this news. A month earlier, a scientific breakthrough was announced referring to a technology which can lead to a much more efficient development of thousands of vaccines and treatments for diseases in the future. This breakthrough was not only relevant for life sciences and biology, but also for Artificial Intelligence (AI) and data science.
We’re talking about DeepMind’s major breakthrough in their latest version of ‘AlphaFold’. AlphaFold is an AI-based system that has been acknowledged as a valid solution to one of the most challenging problems in the field of biology: the problem of “protein folding”. What is the “protein folding” problem and how was it solved using AI?
What is the problem?
Proteins are highly complex substances that are present in all living organisms. They are essentially the building blocks of life. Almost every function in our body relies on proteins and how they change and move. The function of a certain protein depends on its unique shape and structure. For example: the antibody proteins in our immune system have the shape of a catapult, which serves as a hook to latch onto bacteria and viruses to detect and indirectly eliminate them.
How these proteins are built is encoded in our DNA. A fault in the genetic building recipe can result in a misshapen protein, which can lead to diseases. However, just knowing the genetic structure of a protein isn’t enough to give away its shape (which is so important!). Proteins consist of a sequence of amino acids, and the DNA only holds information of this sequence, not how it folds into a unique shape. The larger a protein, the more complicated it is to model its shape. In fact, it would take longer than the age of our universe to randomly try to find the true shape of a protein. Predicting how these chains of amino acids fold to form the 3D structure of a protein is what we call the “protein folding problem”.
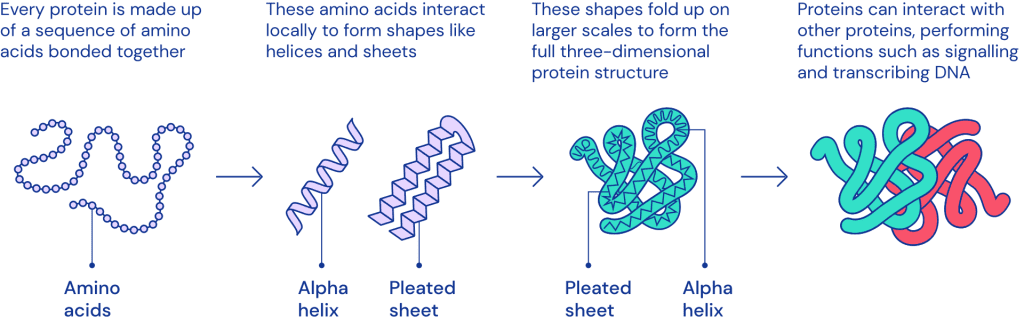
This 50-year old problem has proven itself to be very difficult to solve, which is why the Critical Assessment of Protein Structure Prediction (CASP) competition was created in 1994. Every year top research teams from around the globe attend this competition to present their prediction models in an attempt to solve the protein folding problem. The level of accuracy that is required to solve the problem had not yet been reached. However, in recent years of the competition, Artificial Intelligence has marked its presence and has proven itself to produce groundbreaking results.
Why is it important?
Up until now, the best way to find the 3D structure of a protein was through expensive and time consuming methods. A single protein’s structure takes about a year to find and costs about $120.000 using X-ray crystallography. Besides this method of X-ray crystallography, there isn’t a straightforward analytical way to find the 3D structure of a protein based on its amino-acid sequence. All methods perform way below the bar that DeepMind’s AlphaFold has set in the most recent CASP editions.
AI-methods can be the solution to this time-consuming and expensive process. To be able to predict a protein’s shape from its sequence, can immensely accelerate research. Once the shape of a protein is found, its function can be determined and scientists can develop vaccines and drugs that work with the protein’s unique shape and cure or prevent diseases faster.
DeepMind’s Solution
There is a lot of data available in the field of genomics (the study of all of a person’s genes). This enables approaches using Artificial Intelligence to use this genomic data to solve this problem. The core of any AI-algorithm is essentially data!
DeepMind’s first solution to the protein problem (back in 2018) focussed specifically on modeling the target shapes of the proteins from scratch, where they did not use previously solved proteins. See it as teaching a child to spell a word, without showing them any references of how similar words are spelled.
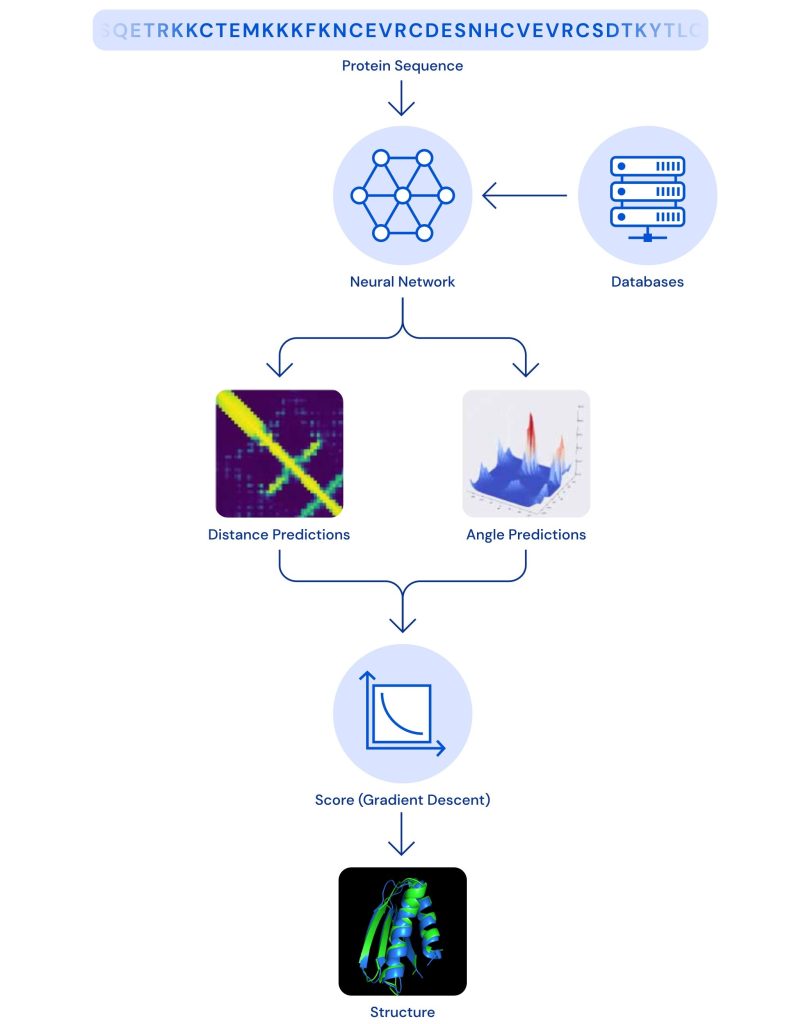
The first AlphaFold system solves the problem in two steps. The first step is predictive modelling using A Convolutional Neural Network (CNN) A CNN is suited particularly well for this, because it can comprehend a highly dimensional input (such as an extremely long protein sequence). CNN’s are also used a lot for analysing images, where you have the same problem of high dimensionality in the amount of colours that could be in an image (your computer can display 16.8 million colours!).
The model inputs the protein sequence and the aim is to output a correct fold. The network predicts two properties: 1) the distance between pairs of amino acids and 2) the angles between chemical bonds that connect those amino acids[1].
The second step optimises the output using a technique called gradient descent. This is a mathematical technique which is used to make small, increasing improvements to the model.
The New Approach
The groundbreaking solution that was announced just a few months ago, was the second version of the already-groundbreaking first AlphaFold system. Not a lot has been published about this new approach besides a single blog post. It seems like the DeepMind team has made two major updates to the system. The first one sparks our interest particularly, because the CNN that they used in the first version has been replaced by a so-called ‘Transformer’: a new deep learning model that was introduced in 2017. A Transformer is an ‘attention-based’ neural network model which can be seen as the successor of the LSTM and CNN. DeepMind took advantage of this recently developed deep learning approach, which enabled them to achieve this major breakthrough.
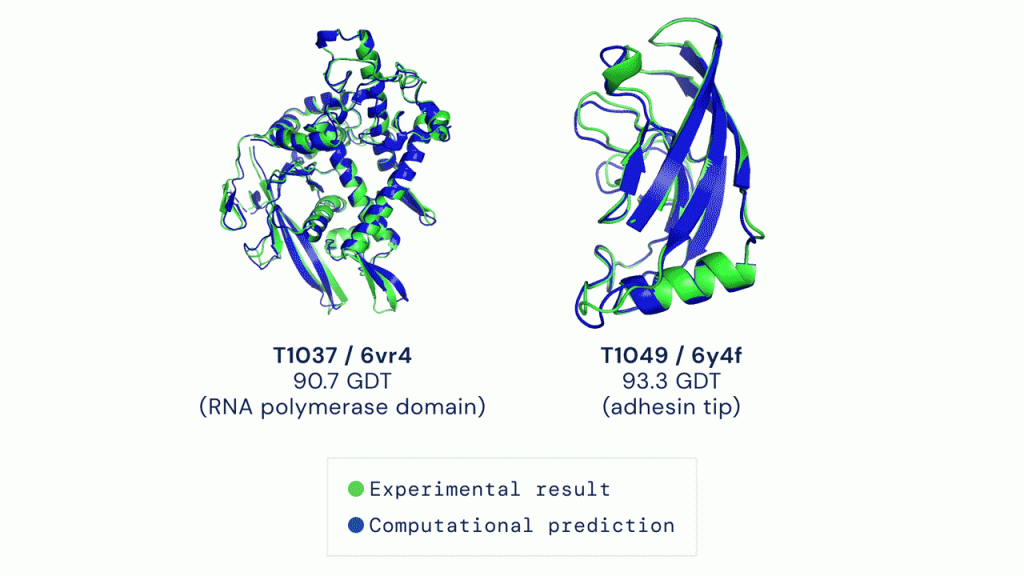
The role of AI
An AI-based solution like DeepMind’s AlphaFold can help scientists acquire more knowledge and eventually contribute to more efficient drug and vaccine discovery. This means that AI can be really useful in research for curing and preventing diseases, and eventually improve the quality of life for many people all around the world.
We have seen the success of the solution of protein folding, and believe this indicates how AI can use various sources of information which help people to find innovative and creative solutions to problems that may seem unsolvable at first. AI-based solutions can enrich our human capabilities for solving complex issues in a way that we have never seen before. We have seen that AI has mastered many games such as AlphaZero and Libratus, and in the same way we believe that AI can help humans in advancing even more by contributing to solving fundamental problems.
We’ve seen how AI can cultivate the world of scientific discovery and its promising potential to improve our quality of life. Many people can benefit from AI-based solutions and these technologies have never been this accessible before. In fact, you are on the right page to become part of the promising and exciting future or AI. Its capabilities are endless and it has so much to offer!