Smart with Sensors
Ensuring safety with AI
Construction sites are obligated by law to measure the amount of inhalable particles, or ‘particulate matter’, at their construction sites to ensure the safety of local residents. However, this requirement comes without solid knowledge of how many sensors need to be used or where they need to be placed. Just to be sure, extra sensors are often placed to avoid mis-measurements. However, these sensors are expensive, and each site used about 20 sensors, resulting in a lot of locked up capital and maintenance costs. Our client saw potential in combining data science with sensor use to find a definitive answer on how many sensors are actually needed and where these should be placed to ensure optimal safety at the highest rate of efficiency.
Optimizing sensor placement
Our main goal was to discover whether data science could be used to optimize the sensor use and placement at construction sites, and to provide our client with actionable insights and working model that can be reused in future construction sites. Our subgoals were to explore the data collected from the sensors, build and test different predictive models, evaluate the performance and accuracy of the models, and determine the optimal number and placement of sensors.
To achieve our goals and solve the challenges our client faced, we took the following actions:
- Data evaluation and preparation: We started by evaluating the quality, completeness, and relevance of the data that our client had collected from 20 sensors at two of their sites. We discovered that there were some gaps in the data, and that we needed to fill these gaps with data interpolation. We also had to adjust the data structure and framework to make it suitable for our analysis and modeling.
- Model development and testing: We experimented with different models, including traditional AI methods, such as cluster algorithms, and intelligent cluster algorithms, to find the best model for the job. We also used data interpolation and extrapolation to fill the gaps and make the data more complete and accurate. We faced a challenge in terms of computational power, but were able to optimize the compute performance and reduce the time needed for analysis.
- Data visualization and presentation: We developed a tool that helped visualize the findings of the data, including graphs that showed the emission density, which sensors picked up what data, and where they had to be placed for the most accurate results. We bundled our findings in a presentation to ensure that the facts were presented in an optimal and clear way, and to enable knowledge transfer.
Getting the job done
We were able to show that data science could be used to optimize sensor use and placement at our client’s sites, and to provide them with actionable insights and recommendations. Some of the key results were:
- We built and tested a predictive model that could estimate the levels of particulate matter at any given location and time, based on the sensor data and other variables, such as weather, traffic, and wind. The model had a high accuracy and performance, and was able to comply with the regulatory standards and the actual measurements.
- We determined the optimal number and placement of sensors that would provide the most reliable and cost-effective results, and visualized the trade-offs and benefits of different scenarios. We showed that up to 20% of costs could be saved on optimal placement, with highly similar measurements. With more data, this number could have been dropped even further.
- We visualized the findings of the data, and made the data insights more understandable and actionable for our client and other stakeholders. The tool also allowed our client to explore different scenarios and see the effects of changing the sensor number and placement on the results.
- We provided our client with a report that summarized our findings and recommendations, and presented our results to them and other relevant parties.
Relevance for Other Companies
The approach and methods we used in this project can be applied to other companies and industries that use sensors and want to optimize their use and placement. Some examples include:
- Manufacturing: Data science can be used to analyze sensor data from production processes to identify inefficiencies and optimize operations. For example, a manufacturing company could use data science to analyze the temperature and humidity levels in their factory to optimize the production of a specific product.
- Energy: Data science can be used to analyze energy usage data to identify patterns and optimize energy consumption. For example, an energy company could use data science to analyze the energy usage of a specific building to identify areas where energy is being wasted and make recommendations for improvements.
- Transportation: Data science can be used to analyze data from vehicle sensors, GPS data, and traffic data to optimize fleet operations. For example, a transportation company could use data science to analyze the routes taken by their trucks to identify areas where they could save time and fuel.
Learnings for Your Organization
One of the most important takeaways from this project is the importance of getting started with AI and data science. Only by doing, we were able to discover the type of measurements needed to create valuable insights and cost effective measurements. With a minimal time spent on data analysis and data collection, you can drastically reduce operating costs and increase efficiency. Additionally, AI can be a great benefit, as it helps you get insight into the quality of your measures and you can rest assured that you are measuring the right thing. By working with us, our client was able to discover the value of data science and how it can benefit their organization, and we can help you do the same.
How we optimized sensor placement for construction sites, reducing costs up to 20% and ensuring regulatory compliance through predictive modelling.
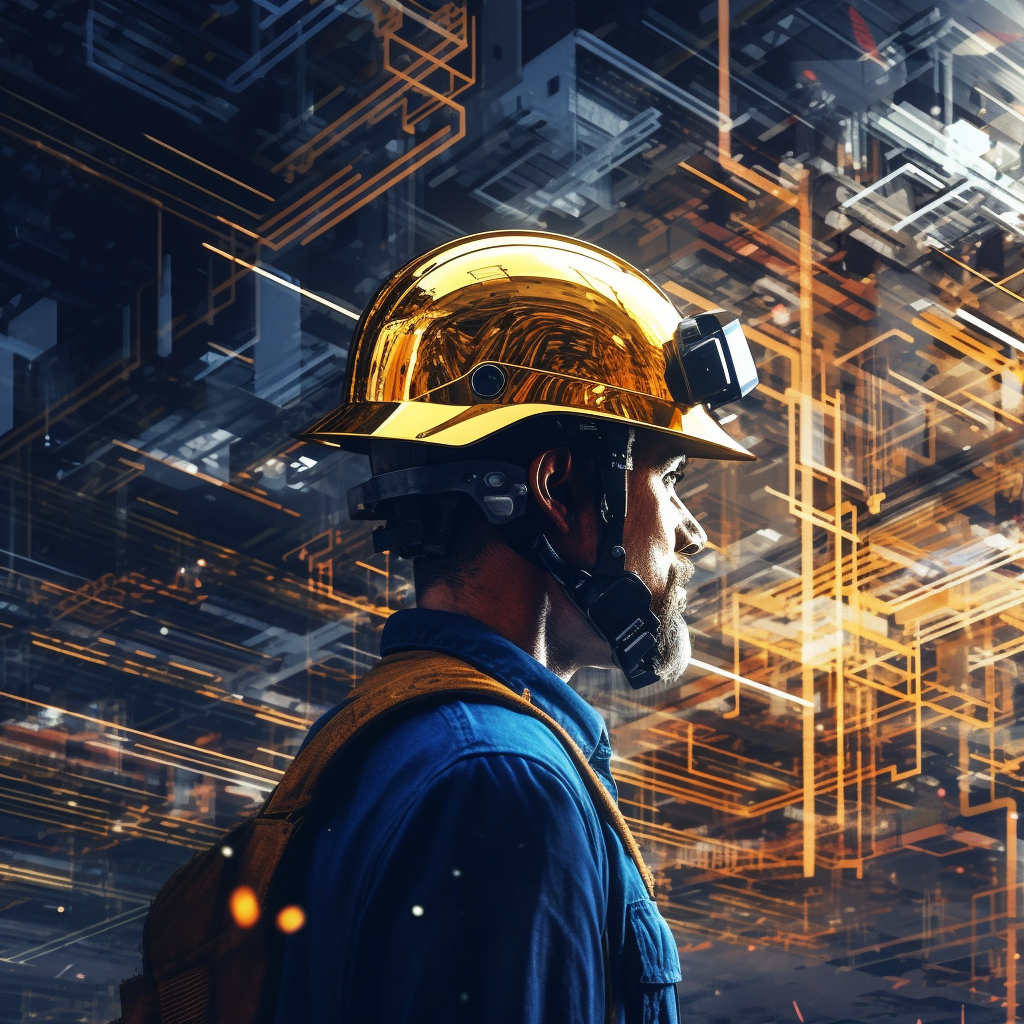